Join our Core Tools II - SPC (Statistical Process Control) and MSA (Measurement Systems Analysis) training, led by expert instructors who are core tools practitioners in today's manufacturing environment.
What exactly is the NDC in MSA? We’ve been asked this question a few times lately so thought it would be a pertinent point to post!
When conducting statistical data analysis you need more than just the data generated by the manufacturing process [the Part Variation (PV)], you also need to be sure of the trustworthiness of the measurement process. A Measurement System Analysis (MSA) calculation explores how much variation in results comes from the measurement equipment (e.g. scales, calipers, micrometers, etc) and other factors (such as the people doing the measuring). The purpose of MSA is to use the results obtained to refine a production process and eliminate errors, as it relates to quality control.
The ndc is one of the criteria used by the MSA to determine the sensitivity of the measuring equipment. It indicates the “Number of Distinct Categories” that can be clearly distinguished by the measurement system being used.
The formula for calculation is NDC = 1.41*(PV/GR&R)
Where:
PV = Part Variation – the real variation of the parts manufactured
GR&R = Gauge Variation – the variation of the measurement process
Gauge Repeatability refers to the ability of a single operator (often a person) to get the same result repeatedly; Gauge Reproducibility refers to the measurements of multiple operators falling within a tight range.
Note that the GR&R measures only the variability in measurements – it does not reveal anything about their accuracy, which can only be assured through calibration, so if inaccurate (junk!) measurement data goes into the calculation in the first place, you’ll get useless results to work off of.
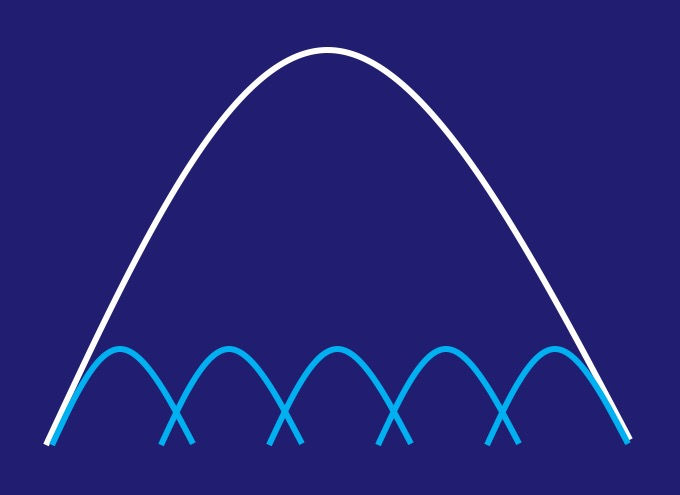
Statistically speaking the ndc is the number of non-overlapping 97% confidence intervals that will span the expected PV. In other words, it represents the number of groups within your product or process data that your measurement system can discern. In layman’s terms, using this graphical representation, the ndc tells you how many times the GR&R curves fit within the PV curve – the more times the better i.e. the more sensitive your measurement equipment is.
How to interpret the results of the ndc calculation:
The resulting value tells you if the measurement equipment is sensitive enough. The higher this number, the better chance the tool has in discerning one part from another, and the guidelines for interpretation are listed below:
ndc ≥ 5 – acceptable measurement equipment
ndc < 5 – rejected measurement equipment - more sensitive equipment needs to be used
ndc < 2 is considered too low to interpret because there is nothing to make comparisons between
ndc = 2 – this is a suboptimal result as the data can only be divided into two groups (high-level and low-level data)
ndc = 3 – this is also a suboptimal result as the data can only be divided into 3 groups, say low, middle, and high
What actions should be taken for ndc scores of < 5?
To increase ndc you need to increase your part variation (PV), decrease the measurement variation (GR&R), or both.
Switching from a caliper to a micrometer does decrease GR&R, but it may not have much of an effect if the PV is small.
If you used very similar parts in your study you may need to re-conduct the study using a true representation of PV, not simply ideal parts. You need to ensure that the parts selected for measurement represent the full spectrum of variation.
Increase the precision of your measurement tools.
Join our Core Tools II - SPC (Statistical Process Control) and MSA (Measurement Systems Analysis) training, led by expert instructors who are core tools practitioners in today's manufacturing environment.
ENCONA provides training and consulting services to the manufacturing industry, via our global network of experienced industry professionals and subject matter experts.
For questions don't hesitate to get in touch with us on enquiries@encona.org
REFERENCES: AIAG Measurement System Analysis Reference Manual – 4th edition
Comments